By Naomi S. Baron (ΦBK, Brandeis University)
Professor of Linguistics, American University
. . . . . . . . . . .
Today’s word on the street is AI. Artificial intelligence. You can’t escape it in the news. Your real estate agent and radiologist boast using it. Depending upon whom you believe, it will be the savior or death star of democracy, even civilization.
In simplest terms, AI is use of computers to perform human cognitive activities. Think of solving a math problem, writing an essay on Madame Bovary, translating that work into Italian, or rendering a visual likeness of Emma Bovary by automatically extracting descriptions from Flaubert’s novel. These days there is also much talk of AGI—artificial general intelligence—meaning the ability of computers to tackle all feats of human intelligence. While AGI doesn’t yet exist, companies like OpenAI are trying.
The lineage of AI dates back before technology existed for building it. In 1948, Alan Turing had thrown down the gauntlet in an unpublished paper, “Intelligent Machinery.” His task: figuring out “whether it is possible for machinery to show intelligent behavior.” The manuscript ended with a hypothetical experiment and equally hypothetical machine, for which the aim was to see if a chess player could determine if he was playing against a human being or computer. The seeds of the famous Turing test for language were sown.
Mathematicians, engineers, and the early generation of computer scientists got to work. The name “artificial intelligence” took root at a 1956 summer working conference at Dartmouth College. An unfolding of new programming models (and ever-better computers) marked the coming years. Much effort focused on human language, especially machine translation, but there was also progress in areas ranging from expert systems and robotics, to graphics and games like chess. Sci-fi movies notwithstanding, the fruits of these initial forays weren’t overwhelmingly impressive—or scary.
The pace picked up sharply in the 2010s. Geoffrey Hinton, along with several of his University of Toronto graduate students, crushed the competition in the 2012 ImageNet contest, an event challenging computers to recognize digital images. The winners’ secret sauce was combining a new programming technique called convolutional neural networks with Nvidia’s graphic processing chips.
A cascade of developments soon followed: transformer architecture, large language models, the first iterations of OpenAI’s GPTs (generative pretrained transformers), ChatGPT, and then GPT-4. Meanwhile, the same powerful GPTs (now often called “foundation models”) proved adept not just at maneuvering written language, but also at spinning out computer code, creating artwork, handling business logistics, and identifying potential new viruses and medicines. Unfortunately, they could also spout gender and racial bias, hallucinate, threaten human employment, and beget social media havoc.
But AI’s potential for good can be breathtaking as well. Take the biomedical sciences. It’s critical to deploy all the arrows in our quiver when the stakes for human health are high. Enter Demis Hassabis, cofounder of the AI company DeepMind (now owned by Google). Hassabis grew up as a computer game aficionado, then earned an undergraduate degree in computer science and a PhD in cognitive neuroscience. DeepMind garnered public notice in 2016 when its program AlphaGo bested a Korean Go champion.
However, the AlphaGo project wasn’t really about games. Hassabis’ deeper interest was in tackling how to untangle the structures of human proteins, a task that has proven devilishly hard for decades. The AI programming approach that Hassabis and his colleagues developed for training AlphaGo enabled them to fast-track the protein decoding process. The payoff: addressing medical challenges from cancer to the next pandemic.
In the humanities, the scorecard is muddier. Think of all the editing and autocomplete tools we take for granted: spellcheck, grammar check, predictive texting. Newer souped-up versions caution (sometimes mistakenly) that our language is verbose or not sufficiently inclusive. And, of course, with the launch of ChatGPT, we marvel at AI generating large swaths of text from a brief prompt.
Time and effort are saved. But are we still motivated to learn to spell or to expend the mental labor needed to puzzle through what we want to express—and how? In support of “yes” answers, here are two time-honored writing lessons to heed. Lesson one: Often we don’t know what we think about an issue until we undertake to write about it. In Joan Didion’s words, “I write entirely to find out what I’m thinking.” And the second lesson, this time from Robert Graves: “There is no such thing as good writing, only good rewriting.” The hitch with AI is that if a computer program writes for us, we forgo opportunities for figuring out what we’re thinking. Plus, research suggests that when we hand over drafting to a program like ChatGPT, we tend to be content with the output, giving ourselves a pass on the opportunity to rethink and rewrite.
There’s yet another piece to the AI text generation story. For today’s AI not only writes, it also “reads.” By that we mean it works through text in its massive dataset (including, say, all the works of Shakespeare and of the Greek dramatists), and then responds to our initial writing prompt—maybe to compare Lear and Oedipus as tragic figures. No surprise, AI can also “read” a scientific manuscript and churn out an abstract or scholarly review. Academics get the day off, legitimately or not.
When we let AI do our reading-plus-writing, we rely on programs to interpret and to make judgments for us. At the 2023 Frankfurt Book Fair, Salmon Rushdie sagaciously declared, “I don’t like books that tell me what to think. I like books that make me think.” Just as we lose out when allowing human authors to dictate our ideas, letting AI do our reading and sense-making disenfranchises us.
As scholars and teachers, humanists and everyday citizens, we will need to make our way through the wonderland and minefield of AI. When it comes to human activities such as creating and critiquing, we flesh-and-blood mortals must remain the deciders as to how much power we are willing to cede to the machine. Invoking Nancy Reagan, we get to choose when to “Just say no.”
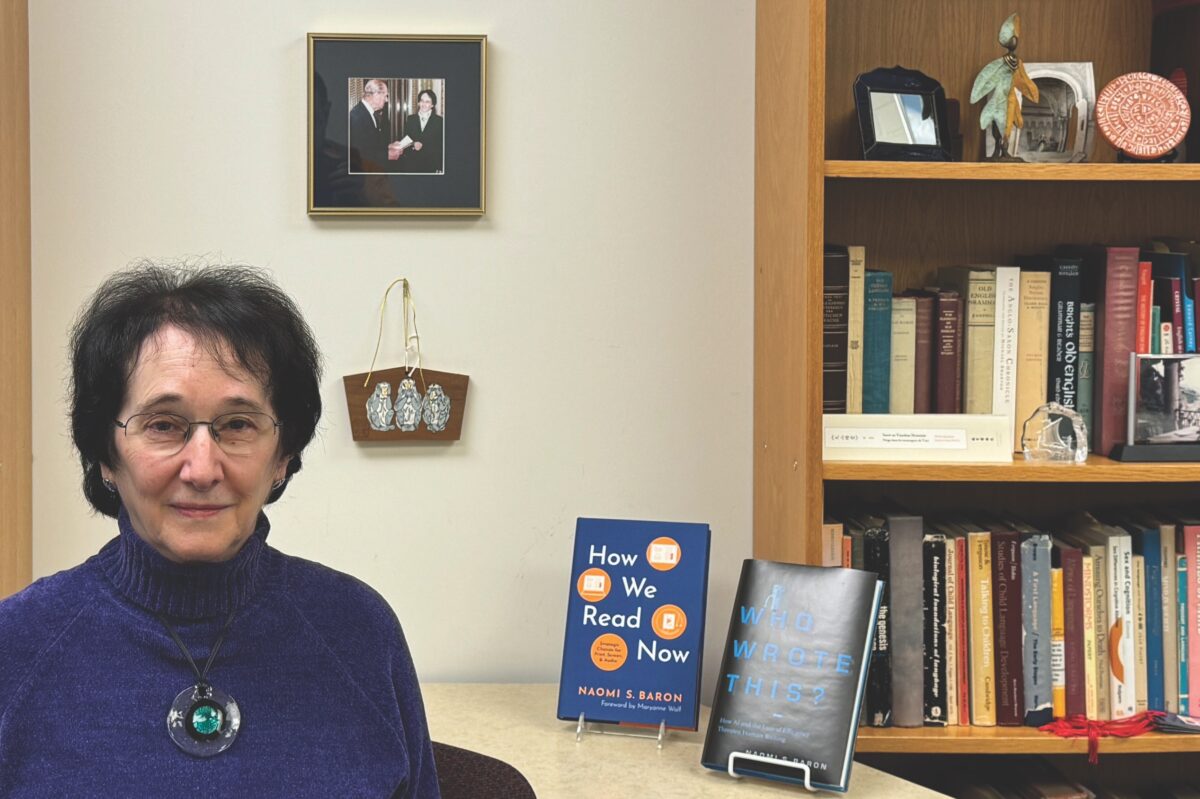
Naomi S. Baron specializes in language and technology. She is the author of Who Wrote This?: How AI and the Lure of Efficiency Threaten Human Writing (Stanford University Press, 2023) and How We Read Now: Strategic Choices for Print, Screen, and Audio (Oxford University Press, 2021).